Could machine learning one day preside over court proceedings, determining facts and outcomes in lieu of a Judge, predicting what would have been contested evidence?
Understanding what identifies Machine Learning, as distinct from Data Analytics and Predictive Analytics, is a useful starting point before going on to explore the capability of the technology, in business and in the wider world. In this brief article I’ll explore some existing and some possible future applications of Machine Learning and, in particular, how it might infiltrate legal proceedings.
The distinction:
In a recent Forbes article, Eric Vardon wrote this neat summary:-
‘AI comes in many shapes and forms. It also has mountainous benefits for companies in 2020. Two of the main types of AI to adopt are machine learning and predictive analytics. The former uses supervised rules and settings to produce a specific outcome. It learns the best next action or opportunity by continually analysing incoming data. Alternatively, you may opt for unsupervised machine learning once your organisation is experienced enough with it. Predictive analytics, on the other hand, is a technology used to predict how marketing, finances and other components of business will perform. This option allows a company to forecast whether or not their efforts will generate a return, next best actions and budget allocation. Companies with large amounts of historical data will find it the most beneficial.’ Machine Learning vs Predictive Analytics: Which is Better for Business - Forbes June 2020
In other words, data analysis is the process of reviewing data from past events for patterns, predictive analytics is then the process of making assumptions based on that historical data to predict future outcomes. Machine learning, meanwhile, analyses current (or incoming) data (together with the data that has already been collected), makes assumptions, learns and provides predictions based on that process (all at a scale and depth not possible for mere mortals).
Back in the summer of 2019 three Netherlands based academics published a research piece entitled ‘Using machine learning to predict decisions of the European Court of Human Rights’ (https://link.springer.com/article/10.1007/s10506-019-09255-y). For me it conjured up a vision of a dystopian nightmare, not unlike the world inhabited by Tom Cruise in Minority Report (a film released in 2002). Crimes were predicted before they had even been contemplated by the future perpetrator, let alone committed, and the would-be offender was punished for their not-yet-happened wrongdoing. My question posed at the beginning of this article is, of course, several steps further back than the process envisaged within Minority Report – focusing on whether Machine Learning could have the capability to one day evaluate all sources of evidence (as if in the position of judge or jury) and determine the outcome of what would have been a contested trial, without the need for the current adversarial process involving lawyers and cross examination. In fact, the research piece turned out to be even further back than that as it acknowledged ‘We are not claiming that were we to encounter a potential victim of a human rights violation, we would be able to predict what the decision in their case would be. While our research is aimed at getting closer to that goal, this is not the purpose of the present study.’ So what was the study and what was the result?
The ambit of the research was defined as follows:
‘The goal of this study is to create a system, which is able to automatically predict the category (i.e. a verdict) associated to a new element (i.e. a case). For this task we will employ machine learning. More specifically, we will use supervised machine learning. In this type of approach the computer is provided with (textual) information from many court cases together with the actual judgements. By providing many of these examples (in the so-called ‘training phase’), the computer is able to identify patterns which are associated with each class of verdict (i.e. violation vs. no violation). To evaluate the performance of the machine learning program, it is provided with a case without the judgement (in the ‘testing phase’) for which it has to provide the most likely judgement. To make this judgement (also called: ‘classification’) the program uses the information it identified to be important during the training phase.
To illustrate how supervised machine learning works, let’s imagine a non-textual example. Suppose we want to write a program that recognises pictures of cats and dogs. For that we need a database of images of cats and dogs, where each image has a label: either cat or dog. Then we show the system those pictures with labels one by one. If we show enough pictures, eventually the program starts recognising various characteristics of each animal, e.g., cats have long tails, dogs are generally more furry. This process is called training or fitting the model. Once the program learns this information, we can show it a picture without a label and it will guess which class the picture belongs to.’
Interestingly, the ‘predicting the future’ experiment was conducted against only three classes of cases; those concerning possible violations of Articles 3 (Prohibition of torture), 6 (Right to a fair trial) and 8 (Right to respect for private and family life), as these made up the datasets with the largest amounts of cases.
The results:
When seeking to predict outcomes only on cases from specific years (2014-2015 and 2016-2017) referable to the training datasets (cases up to and including 2013) the algorithm had a success rate of 66% for 2016-2017 cases, rising to 68% for 2014-15. This increase can be attributed to the proximity of the time period to the training data. This is a conclusion supported by the fall in successful prediction to 58% when the 2016-2017 cases were analysed against a training data set which included cases only up to 2005. When predicting the outcome for a random selection of cases (rather than specific years) the success rate increased to 77% accuracy. The conclusion was this, ‘predicting future judgments is indeed a harder task, and it gets harder if the gap between the training and testing data increases’. Whilst the authors acknowledge the Machine Learning model adopted was ‘very simple’ and that they hope to better predict court judgments by using more advanced machine learning techniques, for the moment, at least, it seems lawyers and judges will remain in gainful employment.
But let’s return to the example of the cat and dog used by the authors of the research to explain how supervised machine learning works, because isn’t this just a very simplified way of explaining AI facial recognition? And isn’t this the same technology that was recently scrutinised in the Court of Appeal decision Bridges, R (On the Application Of) v South Wales Police [2020] EWCA Civ 1058 (11 August 2020)which specifically concerned automated facial recognition (AFR)? In this case the court was considering the lawfulness of the following:
‘When AFR Locate is deployed [the police force] mounts CCTV cameras on police vehicles, or on poles or posts, so as to capture images of the face of anyone who passes within range of the camera… 3 digital images of faces of members of the public are taken from the CCTV feeds and processed in real time to extract facial biometric information. That information is then compared with facial biometric information of persons on a watchlist prepared for the purpose of that specific deployment. The images selected for inclusion on a watchlist will depend on the purpose of each specific deployment.
The watchlists used in the deployments in issue in this case have included (1) persons wanted on warrants, (2) individuals who are unlawfully at large (having escaped from lawful custody), (3) persons suspected of having committed crimes, (4) persons who may be in need of protection (e.g. missing persons), (5) individuals whose presence at a particular event causes particular concern, (6) persons simply of possible interest to [the police force] for intelligence purposes and (7) vulnerable persons. …
AFR Locate is capable of scanning 50 faces per second (although that does not necessarily mean 50 different people). Beyond those technical limitations, there is no limit on the number of persons who may have their facial biometrics captured during any given deployment.
Over the 50 deployments that were undertaken in 2017 and 2018, around 500,000 faces may have been scanned. The overwhelming majority of persons whose biometrics are captured and processed are not suspected of any wrongdoing and are not otherwise of interest to the police.’
This certainly starts to feel like the beginnings of ‘Minority Report’ territory. The challenge in this case was to the lawfulness of the deployment of this type of AFR technology and the Court’s conclusion was that it constituted a breach of Article 8 ECHR (European Convention on Human Rights), namely the ‘Right to respect for private and family life, home and correspondence’. Again, for the moment at least, it seems that thankfully when the capability of machine learning is deployed in a way that offends our Human Rights the Judiciary will impose safeguards on its use.
Continuing this theme the BBC recently published an interesting report entitled ‘The algorithms that make big decisions about your life'. It includes sections on social media, insurance, healthcare and policing. In respect of policing the report says this:
‘Big data and machine learning have the potential to revolutionise policing. In theory, algorithms have the power to deliver on the sci-fi promise of "predictive policing" - using data, such as where crime has happened in the past, when and by whom, to predict where to allocate police resources. But that method can create algorithmic bias - and even algorithmic racism.’
A further worthwhile read for those interested in this area is the Royal United Services Institute for Defence and Security Studies report on Data Analytics and Algorithms in Policing in England and Wales in England and Wales published in February 2020. It’s a heavy read but it’s conclusions are sanguine for those keen to ensure technology is deployed ethically when it effects how we are treated by the State.
Later in September (NobleProg’s dedicated month to focus on all things Data Analytics and Machine Learning) we will be delving into the ethics and bias in machine learning (for which, as a starter, I can recommend also Ethics Guidelines for Trustworthy AI, which includes the following definition: ‘Bias is an inclination of prejudice towards or against a person, object, or position. Bias can arise in many ways in AI systems. For example, in data-drive AI systems, such as those produced through machine learning, bias in data collection and training can result in an AI system demonstrating bias’). This isn’t just a foray into something interesting but irrelevant, the whole point of deploying machine learning in business is to generate reliable predicted outcomes, from which equally reliable decisions can be based. If the process is flawed in any way, the outcomes could be skewed and the reliability of the decisions that flow from that process are seriously or evenly fatally undermined.
Predicting the future is everything in business. If we can do that we can survive and thrive in the face of anything, making accurate decisions along the full spectrum of important decision making activity – from operations to our high level strategy, with ROI and risk analysis properly and fully assessed at every stage.
One of the key challenges for businesses is understanding the different approaches to Machine Learning. In simplistic, metaphoric, terms, it is about asking the right questions to get to the answers that will assist you. In a recent delivery of Machine Learning and Data Science one delegate gave the following succinct feedback:
“I feel I have a much clearer understanding of the processes and techniques used in Machine Learning and when I would use one approach over another. Our challenge now is to practice what we have learned and start to apply it to our problem domain.”
But from a training point of view it’s also about the effectiveness of the pedagogical approach (particularly in an area so easy to become so complex). NobleProg is all about professional skills development, customising courses and delivering knowledge as applied to real world examples, encapsulated (far better than I could) by this feedback following a recently delivered bespoke Machine Learning course:
“[the trainer’s] knowledge of the subject was impressive. He is an absolutely brilliant trainer, able to explain complex concepts in simple language, using practical, real-world examples. Always in a good mood and willing to take time to answer all our questions. He massively improved my knowledge on the subject, and made me feel more confident about machine learning.”
NobleProg’s Machine Learning courses can be delivered via the DaDesktop Training Room – a highly interactive online training platform. Contact the author for a demonstration of this revolutionary technology.
DaDesktop
Your classroom, wherever you want it
Benjamin Jenkins is a Director of NobleProg UK Ltd and NobleProg NL BV, a leading edge technology training and consultancy group. He is also a Barrister who has appeared in multiple reported authorities.
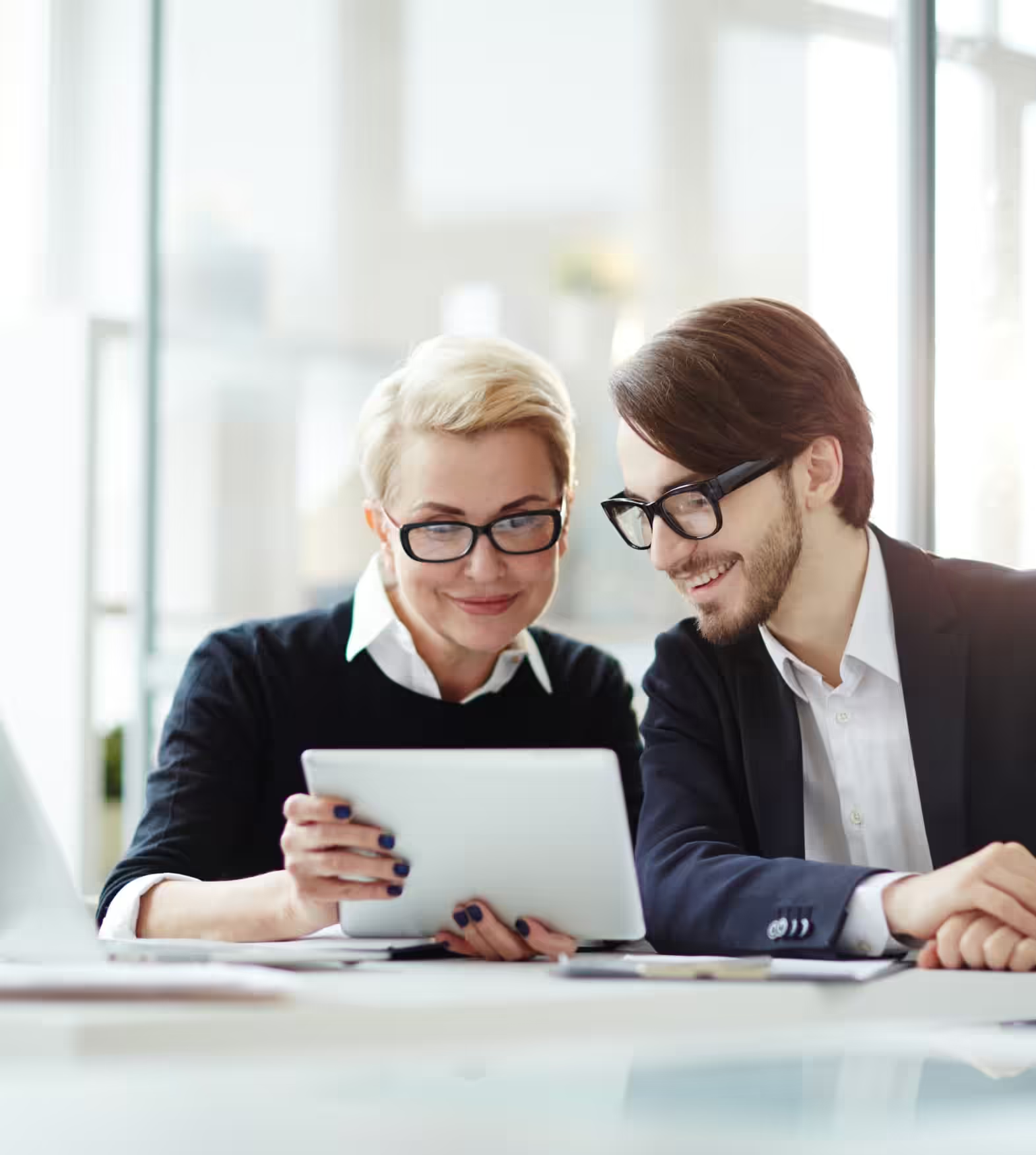
Advies Nodig?
Neem contact met ons op om meer te weten te komen over ons team en de op maat gemaakte oplossingen die wij uw organisatie kunnen aanbieden.
CONTACT ONS